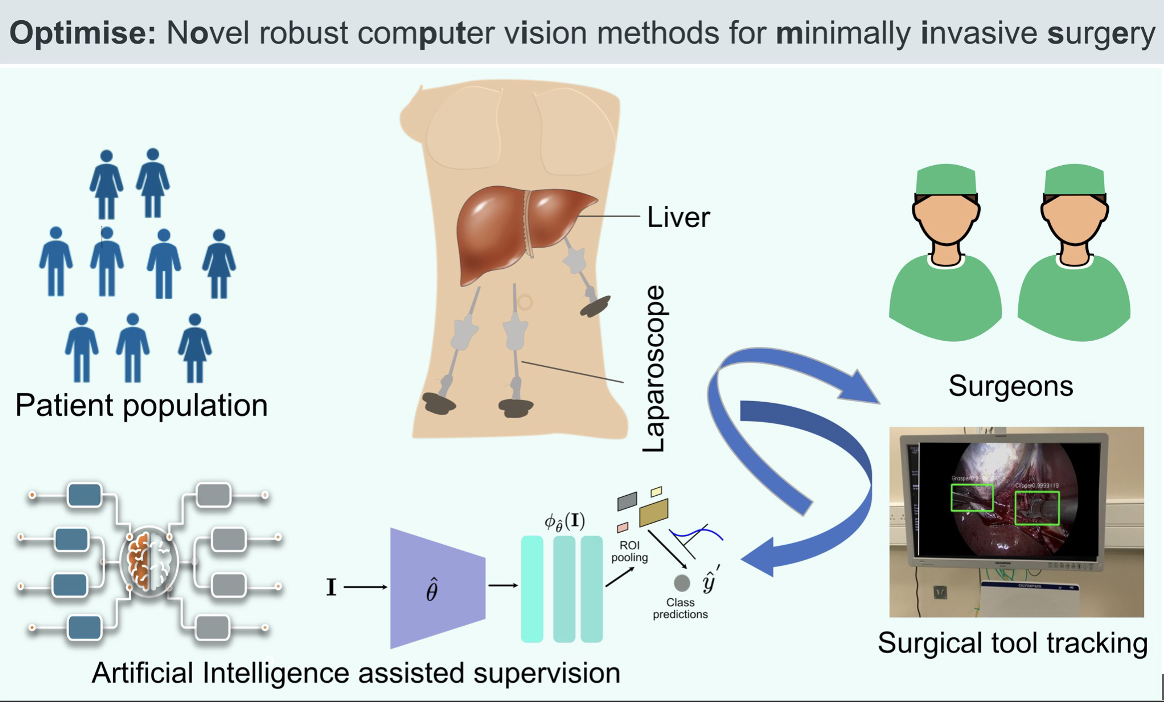
Image-guided Minimally Invasive Surgery (MIS) has grown to be a viable alternative to open-surgery, as it is less painful and leads to shorter recovery times. However, given the state of the current technologies for MIS, such progress comes at the cost of increased complexity, which entails a burden for clinicians, making the procedures prone to errors.
Thus, there is a need to develop novel Surgical Navigation Assistance (SNA) systems which can provide context-aware information about the surgical instruments and the scene to the clinician in real-time. As a matter of fact, accurate and robust tracking of surgical instruments in endoscopy could play a crucial role in MIS, but it remains a challenging task to attain in real-time and in a robust manner using the current state of the art AI models. Therefore, it is necessary to conceive novel computer vision methods for SNA, leading to safer healthcare procedures. For this goal to be attained, novel training strategies need to be conceived; in this project, we will leverage both real and simulated data, as well as novel training strategies for developing more robust SNA systems.
The rapid development in data analysis and processing algorithms offers new avenues for endoscopic data to be used in surgical navigation for multiple purposes, such as in pre-operative planning, intraoperative guidance, and to design post-operative strategies. This new emerging interventional healthcare domain is known as Surgical Data Science (SDS). SDS aims to integrate surgical data with AI tools with the aim of helping to improve the quality of interventional medicine. Furthermore, computer vision (CV) has proven instrumental in several endoscopic-based inspection procedures for the diagnosis of various diseases.
Despite of the great progress that has been made in the endoscopic surgical domain, several challenges remain for applying computer vision in endoscopy: issues like the limited field-of-view, extreme lighting conditions, the presence of image artifacts, a lack of depth information, and difficulty in manipulating operating instruments demand strenuous amounts of effort from the surgeons and need to be addressed to make MIS more efficient and usable.
To this end, the aim of this research proposal is to develop robust and generalizable computer vision methods to provide context-aware real time assistance systems for intraoperative guidance during MIS. A major milestone of our project will be to create new datasets and define metrics and evaluation methodologies for developing computer vision methods for surgical navigation assistance. Our ultimate goal in the long term is to design, test and validate novel deep learning real-time methods that are not only robust to the MIS stringent operating conditions, but that are also able to generalize to multiple types of MIS procedures to make it more usable in a wide range of applications.