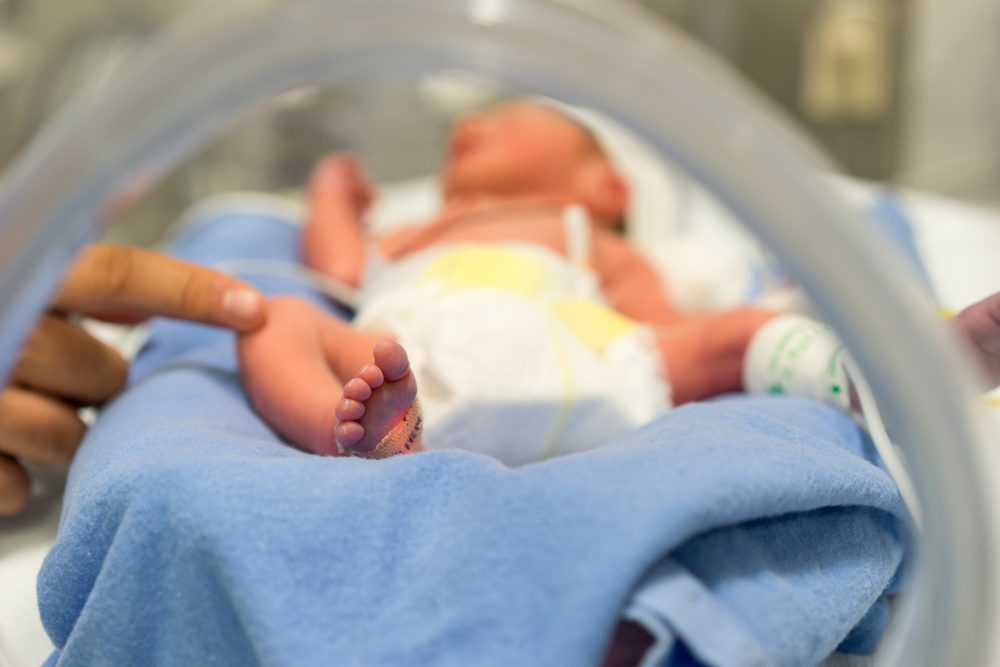
Preterm birth (PTB) is birth before 37 weeks gestation and is the most devastating disease in the perinatal period. One-third of PTB cases are asymptomatic, and clinicians lack useful diagnostic tools to identify these mothers. This diagnostic gap impacts women and babies and impairs our ability to develop treatments for PTB. PTB is linked to maternal chronic stress from adverse childhood experiences, abuse, and intimate partner violence. An individual’s lifelong stress load, including the epigenetic transfer of trauma from previous generations, is their allostatic load (AL). When a women’s AL exceeds her resilience threshold, this may lead to adverse health consequences, including PTB.
We developed an innovative tool using artificial intelligence deep neural networks (DNN) that identifies when asymptomatic pregnant women are at risk for PTB based on their answers to a stress history questionnaire. Our initial model was built and tested on the responses of 135 postpartum participants to the Wellbeing and Pregnancy questionnaire for a retrospective study. Forty-three were cases (PTBs), and 92 were controls (term deliveries). The DNN model was trained on the day of delivery on 20% of the controls and 20% of the preterm deliveries. When the model was tested on the other 80% of the PTB cases, it predicted them all and was off by an average of only 6.5 days (SD = 6.5d) from the actual date of birth.
This study will further test our DNN method in multiple global jurisdictions to determine its efficacy in predicting PTB risk and timing. We will recruit 50 PTB cases and 100 controls postpartum with our team of obstetricians, perinatal scientists, and computer scientists in Edmonton, Canada; Accra, Ghana; two cohorts in Mexico City; Auckland, New Zealand; Southampton, England, and Hong Kong. Our Wellbeing and Pregnancy questionnaire will be administered to participants shortly after delivery. Data will be entered into online data software and analyzed. We hypothesize that our DNN diagnostic tool will predict PTB risk and timing regardless of jurisdiction; however, the model will need to be trained specifically for each jurisdiction to optimize its diagnostic accuracy.
Working with multiple partners from WUN allows us to test the universality of our diagnostic tool in various parts of the world with vastly different cultures and socioeconomic factors. If successful, we will expand our studies in each jurisdiction. Rather than testing the model retrospectively, we will administer the questionnaire to women in their first trimester and see if our DNN models accurately predict PTB risk and timing. Our goal is to create an inexpensive and relatively easy diagnostic for asymptomatic PTB to improve the health outcomes of women and children worldwide.