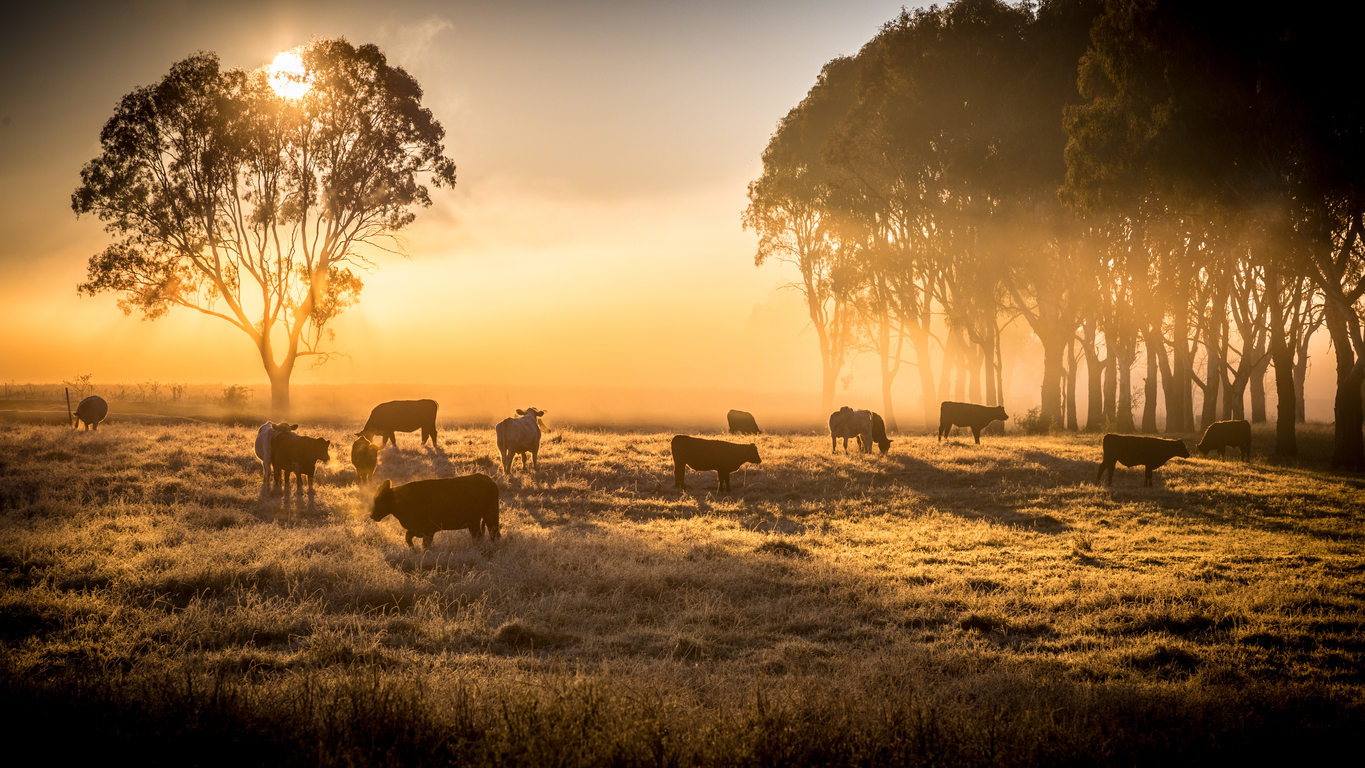
The Research problem
Livestock farming is a cornerstone of global food security, yet climate change is increasingly disrupting pasture productivity and land sustainability. Without timely insights, farmers struggle to manage grazing effectively, impacting both farm resilience and food security. This project focuses on developing an AI-driven sensing model to provide timely monitoring of grazing land conditions using Earth observation technologies. As climate patterns become more unpredictable, pasture growth and availability may fluctuate, threatening livestock farm productivity and sustainability. By analysing the interaction between climate variability and pasture dynamics through Earth observation technologies and advanced machine learning, this research will help farmers and policymakers adapt to future challenges, ensuring sustainable livestock farming and long-term food security.
Research Design
This project aims to develop an AI-driven sensing model to enhance livestock farm management by integrating Earth observation technologies. To achieve this, we will collect data from various sources, including satellite optical and RADAR imagery, multispectral drone surveys, and field measurements of pasture species and land condition. These datasets will be used to analyse pasture dynamics and land sustainability across diverse farm landscapes. The research will focus on pilot farms in Australia, where varying climate conditions provide an ideal setting to test and validate the AI model. The collected data will help us understand how pasture and land conditions change under different environmental factors. Using machine learning algorithms, we will develop predictive models that assess livestock farm productivity and sustainability and provide near real-time insights for management. A key aspect of this research is ensuring the AI model remains explainable and transparent, allowing farmers to integrate predictions into decision-making. By the project’s end, we will generate predictive maps and datasets that enable farmers to accurately monitor farmland conditions. These insights will support adaptive management strategies, ensuring sustainable livestock farming. The outcomes will contribute to climate-resilient food production by equipping farmers with data-driven models to enhance decision-making.
Project Objectives
By the end of this project, we will develop an AI-driven sensing model to monitor and predict pasture and farmland conditions, enhancing livestock farm management and climate resilience. Specifically, this project will (1) integrate multi-source remote sensing products to analyse pasture dynamics and sustainability; (2) develop machine learning models to predict pasture availability and land conditions under varying environmental conditions; and (3) generate spatial-temporal maps that provide actionable insights for optimizing grazing strategies and ensuring long-term sustainability. This project also aims to expand the technology globally by producing transferrable insights applicable to diverse farming systems. By designing a flexible and adaptive AI model, the research will support climate-smart livestock management across various agricultural landscapes. Supported by WUN, this initiative promotes interdisciplinary collaboration among member universities and global partners, driving advancements in sustainable agriculture and equipping farmers with data-driven tools to enhance productivity, resilience, and long-term food security.